Research
FSE IMPACT Fund (Swansea University)
Principal investigator: | Jinlong FU |
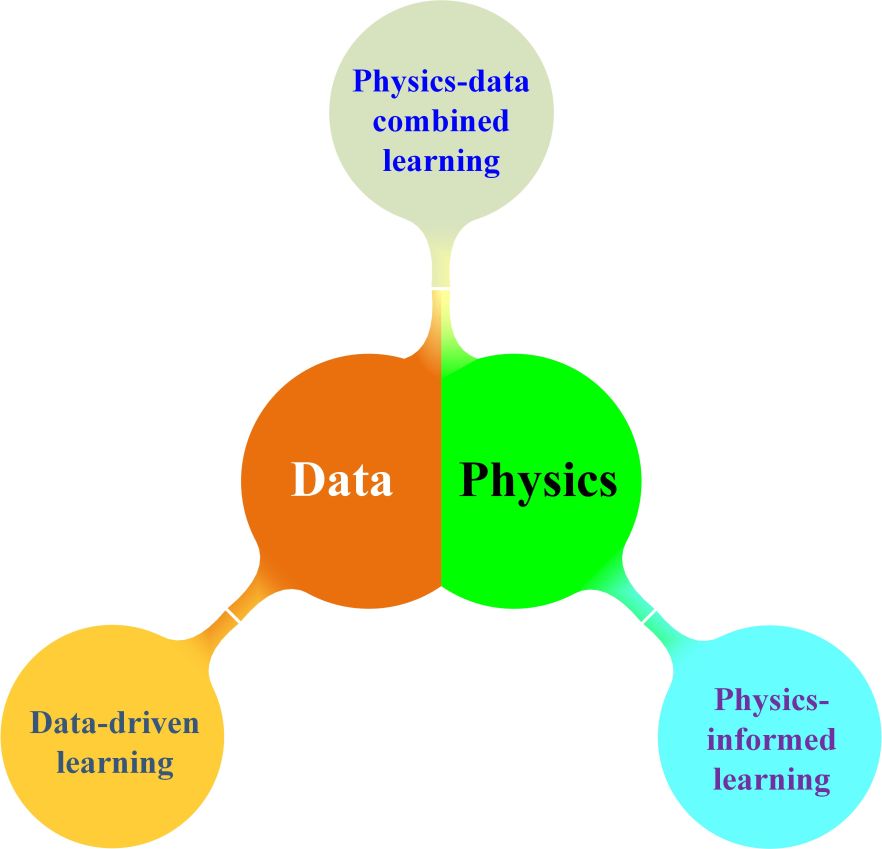
Numerically simulating large-area (urban-scale) turbulent flows in real-time is still a pressing challenge yet to be addressed, because existing 3D CFD urban flow models suffer from an extremely high computational cost. Reduced-order modelling (ROM) provides a means of real-time simulation. Traditional ROM methods such as balanced truncation, proper orthogonal decomposition and dynamic mode decomposition, often restrict the state to evolve in a linear space. In addition, most of the existing ROM methods are data-driven type, so they heavily rely on the available data (high-fidelity snapshots) and are hardly applicable to large-scale problems where both numerical simulation and experimental data are extremely limited.
Physics-informed neural networks offer an opportunity to construct reliable reduced-order models by using known physical principles (such as Navier-Stokes equations) to compensate for the lack of data. Stacked sparse autoencoders will be developed to a new model reduction framework, in which dynamical systems are projected onto a nonlinear space. Deep/machine learning methods will also be used to construct a set of response functions for the parametric and state spaces. Besides, a new data assimilation method will be developed to absorb physical information into data-driven ROM, further increasing model accuracy.
This new ROM framework will provide a practical tool to simulate urban-scale turbulent flows in real-time, such as urban polluted air flows, urban flooding, and tsunami waves hitting coastal cities, which is of great significance for smart city management and risk control.