PhD Research Studentships
Deep learning to model Soft Robot Arms and Hands
Supervisor: | Kaspar ALTHOEFER |
Apply by: | 5 December 2022 |
Description
With the increased interest in the use of soft materials for the creation of highly dexterous robots, soft material robotics has established itself as an important research topic within robotics. Some roboticists predict that soft robotic technologies will play a key role in many areas, such as safe human-robot interaction, minimally invasive surgery, disaster scenarios, underwater and planetary exploration as well as grasping and manipulation of fragile objects.
Although soft robots open up new avenues of application areas especially because of their inherent compliance and capability to safely interact with their environment, controlling their movements to conduct useful tasks is challenging. While it is straightforward to create kinematic models for the more traditional rigid-link robot arms and hands, most analytical models for soft robots are not capable of accurately dealing with the nonlinearities of robot arms made from soft materials and their difficult-to-predict motion behaviour especially when exposed to external forces imposed by interactions with the environment.
This project aims at exploring machine learning techniques, especially deep learning, to generate data-driven models for soft robot arms and hands. Motion data will be acquired from real and simulated soft robot arms and hands conducting a range of movements whilst conducting relevant tasks, such as picking and handling of fragile objects including fruits and vegetables.
Instead of learning neural networks from scratch, this project will make good use of analytical soft robot models (e.g., soft robot kinematic models) and improve on those through the learning approach. Since only aspects that are difficult to model will be learnt, the proposed approach is expected to get more robust training outcomes and require less training. The most important outcomes from the project will be robust kinematic representations and motion primitives for soft robot arms and hands interacting with the physical environment. The joint optimisation of learnt models and the physical robot structures will be attempted.
Funding
This studentship is fully funded* and includes a 3 year stipend (set at £19,668 for 2022/23) and Home tuition Fees.
Eligibility
- Available to applicants with UK Home Fee Status only.*The studentships are also open to international candidates, but they will need to pay the difference in fees between home and overseas. (See: http://www.welfare.qmul.ac.uk/money/feestatus/ for details of UK Home status)
- The minimum requirement for this studentship opportunity is a good Honours degree (minimum 2(i) honours or equivalent) or MSc/MRes in a relevant discipline.
- If English is not your first language, you will require a valid English certificate equivalent to IELTS 6.5+ overall with a minimum score of 6.0 in Writing and 5.5 in all sections (Reading, Listening, Speaking).
- Candidates are expected to start from January 2023
Supervisor
For informal enquiries about this position, please contact Kaspar Althoefer
Application Method:
To apply for this studentship and for entry on to the PhD Full-time Robotics please select Mechanical Engineering - January Start, on the following webpage: Research degrees in Engineering
Please be sure to include a reference to ‘430 SEMS KAA’ to associate your application with this studentship opportunity.
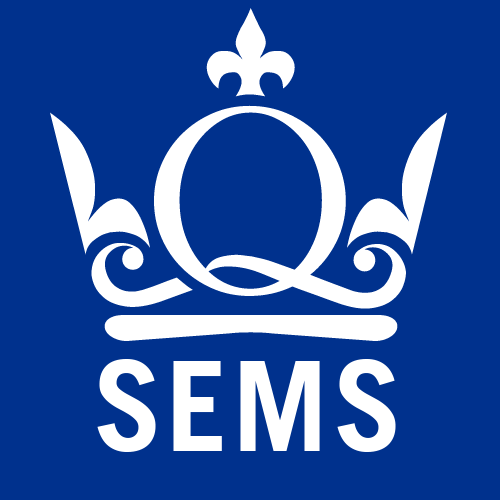
Funding
Funded by: SEMSEligibility
- The minimum requirement for this studentship opportunity is a good honours degree (minimum 2(i) honours or equivalent) or MSc/MRes in a relevant discipline.
- If English is not your first language, you will require a valid English certificate equivalent to IELTS 6.5+ overall with a minimum score of minimum score of 6.0 in each of Writing, Listening, Reading and Speaking).
- Candidates are expected to start in (Semester ).
Contact
For informal enquiries about this opportunity, please contact Kaspar ALTHOEFER.
SEMS Research Centre: |