News
Queen Mary hosts Machine Learning for Combustion Meeting
6 December 2024
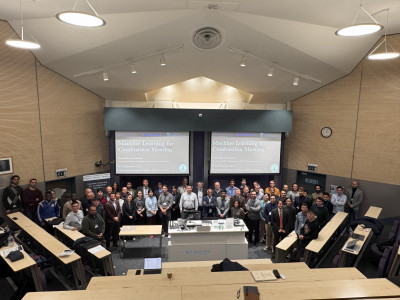
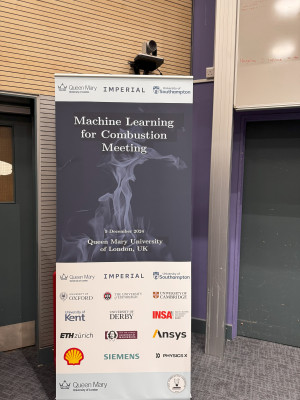
The Machine Learning (ML) for Combustion Meeting was held successfully on 5 December 2024 at Queen Mary University of London. The event saw an excellent line-up of 13 invited speakers, 11 poster presentations and 70 attendees.
The meeting organised under the auspices of Combustion Institute British Section (CIBS) brought together academic researchers and industry professionals to present recent advances in ML for combustion science and engineering. The event highlighted cutting-edge advancements in reactive flow modeling, data-driven techniques, and uncertainty quantification, creating an excellent platform for knowledge sharing and collaboration.
The day began with an insightful keynote by Prof Luc Vervisch from INSA de Rouen Normandie, who shared his perspective on the challenges, successes, and potential of ML for turbulent combustion modeling. The morning sessions covered combustion chemistry acceleration and high-fidelity large eddy simulations leveraging ML. These sessions showcased diverse approaches to integrating machine learning models into CFD solvers and accelerating chemical kinetics calculations.
The afternoon session began with a thought-provoking keynote by Prof Matthew Juniper from the University of Cambridge. His talk emphasised the integration of physical principles as Bayesian priors with quantified uncertainty and demonstrated computationally efficient inference techniques for data assimilation while retaining core physics in modeling. Afternoon sessions focused on topics ranged from probabilistic modeling and alternative fuels to few-shot learning for flame transfer functions and optimising combustion processes through AI.
The vibrant poster session gave early-career researchers a platform to showcase their innovative work, sparking engaging discussions and networking. The day concluded with a panel discussion moderated by Prof Luc Vervisch, where panelists and the audience reflected on the major challenges and opportunities in ML for combustion, setting the stage for future research and collaborations.
A big thanks to all attendees, speakers, poster presenters, and the School of Engineering and Materials Science for their support in hosting this event. Special thanks to our PhD students for their invaluable help in organising and ensuring the smooth running of the meeting.
Contact: | Amin Paykani |
Email: | a.paykani@qmul.ac.uk |
People: | Amin PAYKANI Xi JIANG Chris LAWN |
Research Centres: | Intelligent Transport Sustainable Engineering |